2.3.2 Quantitative Characteristics of Mitigation Scenarios
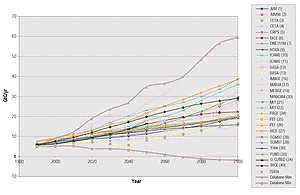
Figure 2.2: Global CO2 emissions from baseline scenarios
used for 550ppmv stabilization quantification (fossil fuel CO2
emissions over the period 1990 to 2100 with the maximum and minimum numbers
of the database of scenarios). This figure excludes the SRES scenarios
(for legend details see Appendix 2.1).
|
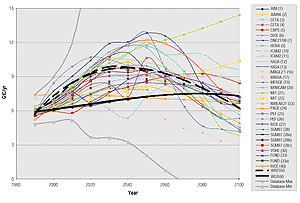
Figure 2.3: Global CO2 emissions from mitigation scenarios
for 550ppmv stabilization (fossil fuel CO2 emissions over the
period 1990 to 2100 with the maximum and minimum numbers of the database
of scenarios). This figure excludes the post-SRES scenarios (for legend
details see Appendix 2.1).
|
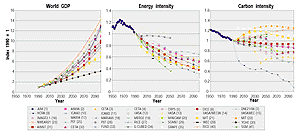
Figure 2.4: Range of baseline assumptions in GDP, energy intensity,
and carbon intensity over the period 1990 to 2100 used for 550 ppmv stabilization
analyses (indexed to 1990 levels), with historical trend data for comparison
(for legend details see Appendix 2.1).
|
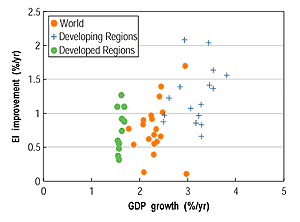
Figure 2.5: Scatter plot of GDP growth versus energy intensity
reduction in baseline scenarios (including world and regional data).
|
From the large number of mitigation scenarios, a selection must be made in
order to clarify in a manageable way the quantitative characteristics of mitigation
scenarios. One of the efficient ways to analyze them is to focus on a typical
mitigation target. As the most frequently studied mitigation target is the 550ppmv
stabilization scenario, a total of 31 stabilization scenarios adopting that
target were selected along with their baseline (reference or non-intervention)
scenarios in order to analyze the characteristics of the stabilization scenarios
as well as their baselines5.
Figure 2.2 shows these baseline scenarios, and Figure
2.3 shows the mitigation scenarios for 550ppmv stabilization. (The sources
and scenario names are noted in Appendix 2.1).
2.3.2.1 Characteristics of Baseline Scenarios
In order to analyze the characteristics of stabilization scenarios, it is very
important to identify the features of the baseline scenarios that have been
used for mitigation quantification. Although the general characteristics of
non-intervention scenarios have already been analyzed in the SRES (Nakicenovic
et al., 2000), more specific analyses are conducted here, focusing on the baseline
scenarios that have been used for 550ppmv stabilization quantification.
First, it is clear that the range of CO2 emissions in baseline scenarios
used for 550ppmv stabilization quantification is very wide at the global level,
as shown in Figure 2.2. The maximum levels of CO2
emissions represent more than ten times the current levels, while the minimum
level represents four times current levels. The range of baseline scenarios
covers the upper half of the total range of the database, and most of them were
estimated to be larger than IS92a (IPCC 1992 scenario a). This means
that the baseline scenarios used for the 550ppmv stabilization analyses have
a very wide range and are high relative to other studies.
This divergence can be explained by the Kaya identity (Kaya, 1990), which separates
CO2 emissions into three factors: gross domestic product (GDP), energy
intensity, and carbon intensity6:
CO2 emissions = GDP * Energy intensity * Carbon
intensity = GDP * (energy/GDP) * (emissions/energy)
Figure 2.4 shows these factors. For comparability of the
factors, which were not harmonized to be the same number among models in the
base year of 1990, all the values are indexed to 1990 levels. CO2
emissions are mostly determined by energy consumption. This, in turn, is determined
by the levels of GDP, energy intensity, and carbon intensity. However, the ranges
of GDP and of carbon intensities in the scenarios are larger than the range
of energy intensities. This suggests that the large range of CO2
emissions in the scenarios is primarily a reflection of the large ranges of
GDP and carbon intensity in the scenarios. Thus, the assumptions made about
economic growth and energy supply result in huge variations in CO2
emission projections.
These characteristics are also observed in regional scenarios. For example,
in both the OECD and non-OECD scenarios, CO2, GDP, energy intensity,
and carbon intensity have wide ranges, and in particular, the range among scenarios
for the non-OECD nations is wider than the range among scenarios for OECD nations.
In addition, the growth of CO2 emissions in non-OECD nations is generally
larger than the growth of emissions in OECD nations. This is mainly caused by
higher GDP growth in the non-OECD countries.
With regard to regional comparisons, it is very difficult to come to any general
conclusions, as the ranges involved in the regional scenarios are extraordinarily
large. Moreover, with the exception of the USA, Europe, the Former Soviet Union
(FSU) and China, the number of available scenarios is limited. However, some
general trends can be identified that are associated with the medium ranges
of the scenarios: for Asian countries, GDP growth is the most significant factor,
resulting in high levels of energy use and CO2 emissions; energy
efficiency improvements are the most significant factor in the scenarios for
China; and carbon intensity reductions are very high in Africa, Latin America,
and Southeast Asia, because of drastic energy mix changes.
Other interesting characteristics at the global level can be identified in
the relationships among GDP, energy intensity, and carbon intensity. Figure
2.5 shows a scatter plot of GDP growth rate versus energy intensity reduction
from the baseline scenarios. As might be expected, the energy intensity reduction
is higher with a higher GDP growth rate, while a lower energy intensity reduction
is associated with a lower GDP growth rate. This relationship suggests that
high economic growth scenarios assume high levels of progress in end-use technologies.
Unlike energy intensity reductions, carbon intensity reductions in the models
are apparently seen as largely independent of economic growth and consequently
are a function of societal choices, including energy and environmental policies.
The scenarios do not show any clear relationship between energy intensity reduction
and carbon intensity reduction. The values depend on regional characteristics
in energy systems and technology combinations. Energy intensity reduction can
include many measures other than fuel shifting. Most of the efficiency measures
will result in lower carbon emissions, and fuel shifts from high-carbon to low-
or non-carbon fuels can increase the efficiency of energy systems in many cases.
However, carbon intensity reductions can also lead to reduced efficiency in energy
systems, as in the case of shifts to biomass gasification or liquefaction, or
result in increased energy consumption, as in the case of industrial carbon sequestration.
Box 2.3. Non-CO2 Mitigation Scenarios
Since the publication of IPCCs SAR, the literature on mitigation
scenarios has continued to focus on the reduction of CO2 emissions
rather than on other GHGs. This is unfortunate because non-CO2
emissions make up a significant fraction of the total basket of
gases that must be reduced under the Kyoto Protocol. However, a
small set of papers has reported on scenarios for mitigating non-CO2
gases, especially CH4 and N2O. In one such paper,
Reilly et al. (1999) compared scenarios for achieving emission reductions
with and without non-CO2 emissions in Annex B countries (those
countries that are included in emission controls under the Kyoto Protocol).
Scenarios that omitted measures for reducing non-CO2 gases
had 21% higher annual costs in 2010 than those that included them. Tuhkanen
et al. (1999) and Lehtilä et al. (1999) came to similar conclusions
in a scenario analysis for 2010, they found that including CH4
and N2O in mitigation strategies for Finland reduced annual
costs by 20% in the year 2010 relative to a baseline scenario. The general
conclusion of these papers is that small reductions of GHG emissions,
for example of the magnitude required by the Kyoto Protocol, can be accomplished
at a lower cost by taking into account measures to reduce non-CO2
gases, and that a small reduction of non-CO2 gases can produce
large impacts at low cost because of the high global warming potential
(GWP) of these gases.
In another type of scenario analysis, Alcamo and Kreileman (1996) used
the IMAGE 2 model to evaluate the environmental consequences of a large
set of non-CO2 and CO2 mitigation scenarios. They
concluded that non-CO2 emissions would have to be controlled
along with CO2 emissions in order to slow the increase of atmospheric
temperature to below prescribed levels. Hayhoe et al. (1999) pointed out
two additional benefits of mitigating CH4, an important non-CO2
gas. First, most CH4 reduction measures do not require the
turnover of capital stock (as do CO2 measures), and can therefore
be carried out more rapidly than CO2 reduction measures. Second,
CH4 reductions will have a more immediate impact on mitigating
climate change than CO2 reductions because the atmosphere responds
more rapidly to changes in CH4 than to CO2 concentrations.
|
|