9.3 Projections of Climate Change
9.3.1 Global Mean Response
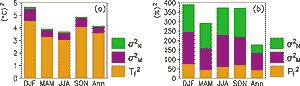
Figure 9.4: Intercomparison statistics for seasonal and annual (a)
temperature and (b) precipitation changes in nineteen CMIP2 experiments
at the doubling of CO2 (years 61 to 80). The total length of
the bars shows the mean squared amplitude of the simulated local temperature
and precipitation changes averaged over all experiments and over the whole
world. The lowermost part of each bar represents a nominally unbiased “common
signal”, the mid-part directly model-related variance and the top part
the inter-experiment variance attributed to internal variability. Precipitation
changes are defined as 100% x (PG-PCTRL) / Max(PCTRL,
0.25 mm/day), where the lower limit of 0.25 mm/day is used to reduce the
sensitivity of the global statistics to areas with very little control run
precipitation. |
Since the SAR, there have been a number of new AOGCM climate
simulations with various forcings that can provide estimates of possible future
climate change as discussed in Section 9.1.2. For the
first time we now have a reasonable number of climate simulations with different
forcings so we can begin to quantify a mean climate response along with a range
of possible outcomes. Here each model’s simulation of a future climate
state is treated as a possible outcome for future climate as discussed in the
previous section.
These simulations fall into three categories (Table
9.1):
- The first are integrations with idealised forcing, namely, a 1%/yr compound
increase of CO2. This 1% increase represents equivalent CO2,
which includes other greenhouse gases like methane, NOx etc. as
discussed in Section 9.2.1. These runs extend at
least to the time of effective CO2 doubling at year 70, and are
useful for direct model intercomparisons since they use exactly the same forcing
and thus are valuable to calibrate model response. These experiments are collected
in the CMIP exercise (Meehl et al., 2000a) and referred to as “CMIP2”
(Table 9.1).
- A second category of AOGCM climate model simulations uses specified time-evolving
future forcing where the simulations start sometime in the 19th century, and
are run with estimates of observed forcing through the 20th century (see Chapter
8). That state is subsequently used to begin simulations of the future
climate with estimated forcings of greenhouse gases (“G”) or with
the additional contribution from the direct effect of sulphate aerosols (“GS”)
according to various scenarios, such as IS92a (see Chapter
1). These simulations avoid the cold start problem (see SAR) present in
the CMIP experiments. They allow evaluation of the model climate and response
to forcing changes that could be experienced over the 21st century. The experiments
are collected in the IPCC-DDC. These experiments are assessed for the mid-21st
century when most of the DDC experiments with sulphate aerosols finished.
- A third category are AOGCM simulations using as an initial state the end
of the 20th century integrations, and then following the A2 and B2 (denoted
as such in Table 9.1) draft marker SRES forcing
scenarios to the year 2100 (see Section 9.1.2). These
simulations are assessed to quantify possible future climate change at the
end of the 21st century, and also are treated as members of an ensemble to
better assess and quantify consistent climate changes. A simple model is also
used to provide estimates of global temperature change for the end of the
21st century from a greater number of the SRES forcing scenarios.
Table 9.1 gives a detailed overview of all experiments
assessed in this report.
9.3.1.1 1%/yr CO2 increase (CMIP2) experiments
Figure 9.3 shows the global average temperature
and precipitation changes for the nineteen CMIP2 simulations. At the time of
CO2 doubling at year 70, the 20-year average (years 61 to 80) global
mean temperature change (the transient climate response TCR; see Section
9.2) for these models is 1.1 to 3.1°C with an average of 1.8°C and
a standard deviation of 0.4°C (Figure 9.7).
This is similar to the SAR results (Figure 6.4 in Kattenberg et al., 1996).
At the time of CO2 doubling at year 70, the 20-year average (years
61 to 80) percentage change of the global mean precipitation for these models
ranges from -0.2 to 5.6% with an average of 2.5% and a standard deviation of
1.5%. This is similar to the SAR results.
For a hypothetical, infinite ensemble of experiments, in which Tm
and T'' are uncorrelated and both have zero means,
{ T2} = Tf2
+ {Tm2} + {T''2} = Tf2+
2M
+ 2N.
The ensemble mean square climate change is thus the sum of contributions from
the common forced component (Tf2), model differences ( 2M),
and internal variability ( 2N
). This framework is applied to the CMIP2 experiments in Figure
9.4. These components of the total change are estimated for each grid box
separately, using formulas that allow for unbiased estimates of these when a
limited number of experiments are available (Räisänen 2000, 2001).
The variance associated with internal variability 2N
is inferred from the temporal variability of detrended CO2 run minus
control run differences and the model-related variance 2M
as a residual. Averaging the local statistics over the world, the relative agreement
between the CMIP2 experiments is much higher for annual mean temperature changes
(common signal makes up 86% of the total squared amplitude) than for precipitation
(24%) (Figure 9.4).
The relative agreement on seasonal climate changes is slightly lower, even
though the absolute magnitude of the common signal is in some cases larger in
the individual seasons than in the annual mean. Only 10 to 20% of the inter-experiment
variance in temperature changes is attributable to internal variability, which
indicates that most of this variance arises from differences between the models
themselves. The estimated contribution of internal variability to the inter-experiment
variance in precipitation changes is larger, from about a third in the annual
mean to about 50% in individual seasons. Thus there is more internal variability
and model differences and less common signal indicating lower reliability in
the changes of precipitation compared to temperature.
|