5.3.4.2. Historical Analogs of Adaptation
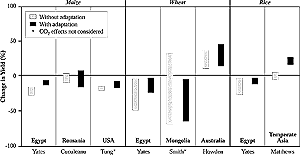
Figure 5-2: Ranges of percentage changes in crop
yields (expressed in vertical extent of the vertical bars only) spanning
selected climate change scenarioswith and without agronomic adaptationfrom
paired studies in Table 5-4. Each pair of
ranges is differentiated by geographic location and crop. Pairs of vertical
bars represent the range of percentage changes with and without adaptation.
Endpoints of each range represent collective high and low percentage
change values derived from all climate scenarios used in the study.
Horizontal extent of the bars is not meaningful. Note that on the x-axis
the last name of the lead author is listed as it appears on Table
5-4. See Table 5-4 for details on climate
scenarios used and types of adaptation strategies modeled in each study.
In the case of Mongolia, adaptation was not modeled when climate change
resulted in positive yield change. In Romania, earlier planting of irrigated
maize results in slightly lower yields than with current planting dates.
|
The agriculture sector historically has shown enormous
capacity to adjust to social and environmental stimuli that are analogous to
climate stimuli. Historical analogs of the adaptability of agriculture to climate
change include experience with historical climate fluctuations, deliberate translocation
of crops across different agroclimatic zones, rapid substitution of new crops
for old ones, and resource substitutions induced by scarcity (Easterling, 1996).
In the Argentine Pampas, the proportion of land allocated to crops has increased
markedly at the expense of grazing land during historic humid periods, and vice
versa during dry periods (Viglizzo et al., 1997). Historical expansion
of hard red winter wheat across thermal and moisture gradients of the U.S. Great
Plains provides an example of crop translocation (Rosenberg, 1982). At present,
the northern boundary of winter wheat in China is just south of the Great Wall
and the north edge of China, where large temperature increases are expected
under climate change (Lin, 1997). Winter wheat planting has shifted from Dalian
(38º54'N) to Shenyang (41º46'N) in Liaoning province. The
shift was aided by introduction of freeze-resistant winter wheat varieties from
high-latitude countries such as Russia, the United States, and Canada into Liaoning
province (Hou, 1994, 1995; Chen and Libai, 1997). Rapid introduction of canola
in Canadian agriculture in the 1950s and 1960s shows how rapidly farmers can
modify their production systems to accommodate a new crop (National Research
Council, 1991). Adaptation to declining groundwater tables by substituting dryland
for irrigated crops in regions of the U.S. Great Plains is an example of substitutions
to deal with water becoming a scarce production resource (Glantz and Ausubel,
1988). None of these examples, however, deals specifically with an evolving
climate change and all are historicwhich limits confidence in extending
their conclusions to future climate change.
5.3.4.3. Agronomic Adaptation of Yields
Increasing numbers of studies have investigated the effectiveness of agronomic
adaptation strategies (e.g., adjustments in planting dates, fertilization rates,
irrigation applications, cultivar traits) in coping with climate-induced yield
losses and gains since the SAR (see Table 5-4).
Considerable costs could be involved in this process, howeverfor example,
in learning about and gaining experience with different crops or if irrigation
becomes necessary. In some cases, a lack of water resulting from climate change
might mean that increased irrigation demands cannot be met (see Section
4.7.2).
Methodologically, there has been little progress since the SAR in modeling
agronomic adaptations. On one hand, the adaptation strategies being modeled
are limited to a small subset of a much larger universe of possibilities, which
may underestimate adaptive capacity. On the other hand, the adaptations tend
to be implemented unrealistically, as though farmers are perfectly clairvoyant
about evolving climate changes, which may inflate their effectiveness (Schneider
et al., 2000). Some studies find agronomic adaptation to be most effective
in mid-latitude developed regions and least effective in low-latitude developing
regions (Rosenzweig and Iglesias, 1998; Parry et al., 1999). This finding
clearly is supported across the studies summarized in Table
5-4, although the number of studies that include adaptation is not large.
A small number of studies in Table 5-4 compare yield
changes with and without agronomic adaptation. Percentage changes in yields
across a range of climate change scenarios for those studies are shown in Figure
5-2. Each pair of vertical bars represents the range of percentage changes
by crop, with and without adaptation, for each study. Clearly, adaptation ameliorates
yield loss (and enhances yield gains) in most instances. The median adapted
yields (mid-point of the vertical bars) shift upward relative to the median
unadapted yields in six of the eight studies. Two studies do not show such an
upward shift (Mongolia, Romania) because of peculiarities in the modeling (see
figure caption). Adaptation ameliorates the worst yield losses in seven of the
eight studies.
It is important to note, however, that differences in modeling methodology
and aggregation of results often lead to conflicting conclusions in specific
regions. For example, in two studies that used the same GCM scenarios, Matthews
et al. (1997) simulate large increases, whereas Winters et al.
(1999) simulate large decreases in rice yield with adaptation across several
countries in Asia (see Table 5-4c). Hence, confidence
in these simulations is low.
Important work has investigated the geographic distribution of crop potential
under climate change. Carter and Saarikko (1996) used crop models to demonstrate
a poleward shift in Finnish potential cereal cultivation by 100-150 km
for each 1°C increase in mean annual temperature. Reyenga et al.
(1999) indicate that climate change accompanied by a doubling of CO2
is likely to enable expansion of existing Finnish wheat-growing areas into dry
margins, considerably extending potential cropping areas. This expansion is
moderated by dry conditions but not effectively enhanced by wetter conditions.
Box 5-5. Extending Uncertainty in Crop Models to Uncertainty in
Economic Analysis
Several factors contribute uncertainty to modeling of
impacts of climate change on agricultural systems (Parry et al.,
1999). Crop modeling studies invariably highlight the need to develop
confidence that the outputs are not "model-dependent." There
is uncertainty because of the fact that yield estimates obtained in
climate impact assessments vary from one impact model to another. In
some cases, simulations across models may exhibit good agreement. Comparison
of rice models showed that their predictions for potential production
were quite close to observed values (Peng et al., 1995). The
Erosion Productivity Impact Calculator (EPIC), a generalized crop model,
predicts observed yields most closely during years with extreme warmth,
lending confidence to its ability to predict yields under climate change
conditions (Easterling et al., 1996). In other situations, agreement
is not as good. In comparisons between wheat simulation models (Goudriaan
et al., 1994; Wolf et al., 1996), grain yield predictions
were markedly different between models. Such findings have stimulated
work to compare the performance of different models and to analyze the
underlying reasons for differences (Gregory et al., 1999; Mearns
et al., 1999).
Spatial resolution of crop models is another important
source of uncertainty in crop models. Crop models simulate processes
that regulate growth and development at fine scales (a few kilometers),
whereas climate change scenarios that drive crop models typically are
produced by climate models operating at coarse scales (1,000 km or more)
(Barrow and Semenov, 1995; Easterling et al., 1998). Studies
that use statistical downscaling techniques and nested limited area
numerical models to increase the resolution of GCM scenarios of climate
change have shown large simulated yield discrepancies between coarse-resolution
(GCM) and fine-resolution (downscaled) climate change scenarios. Mearns
et al. (1999) demonstrate for a site in Iowa that yields can
change algebraic sign depending on the resolution of the climate change
scenario: Maize yield decreases (-11%) with low-resolution climate change
(CSIRO model) and increases (2%) with high-resolution climate change
(RegCM limited area model nested within CSIRO model). Causes of variance
in model output must be identified.
|
5.3.4.4. Adaptation of Livestock
Significant costs can be incurred to counter the effects of climate change
on animal production; moreover, the impact of a warmer climate in terms of costs
is not linear: Larger changes in climate can increase costs exponentially (Hahn
and Morgan, 1999). Possible benefits of climate change during cooler seasons
are not well documented, but the benefits are likely to be less than the consequential
negative hot weather impacts (Hahn et al., 1992). The ability of livestock
managers to cope with climate is demonstrated daily in their coping with normally
varying conditions. A variety of management adaptations are available for livestock
production systems. For example, Hahn and Mader (1997) outline a series of proactive
management countermeasures that can be taken during heat waves (e.g., shades
and/or sprinklers) to reduce excessive heat loads. Historical success in coping
with climate variability suggests that livestock producers are likely to adjust
to climate change successfully. Johnson (1965) provides examples from advances
in genetics and breeding as related to the environment. These capabilities should
allow adaptation to changing, less favorable circumstances associated with projected
rates of climate change. However, coping can entail significant dislocation
costs for certain producers. For individual producers, uncertainties associated
with potential climate change imply additional risks related to how and when
to adapt current production practices (Lewandrowski and Schimmelpfennig, 1999).
Confidence in the foregoing projections of the ability of livestock producers
to adapt their herds to the physiological stresses of climate change is difficult
to judge. The general lack of simulations of livestock adaptation to climate
change is problematic. This absence of a well-developed livestock counterpart
to crop modeling of adaptation assessments suggests a major methodological weakness.
Hence, we give only low to moderate confidence in projections of successful
livestock adaptability.
|